Research Article
Comparative Analysis of Lasso and Bridge Regression Using Corruption Perception Index and Its Correlates in Nigeria
Ogoke Uchenna Petronilla* and Nduka Ethelbert Chinaka
Department of Mathematics and Statistics, University of Port Harcourr, Nigeria
Ogoke Uchenna Petronilla, Department of Mathematics and Statistics, University of Port Harcourr, Nigeria.
Received Date:November 23, 2022; Published Date:January 18, 2023
Abstract
This study compared LASSO and Bridge regression to determine whether the index of corruption perception is influenced by Human Development, Global Hunger, Global Peace and Consumer Price Indices. The best regression technique in model analysis in handling multicollinearity on Corruption perception and its correlates became imperative. A set of secondary data from 2008 to 2020 from Transparency International, World Bank, Knoema and Country Economy on Corruption Perception, Human Development, Global Hunger, Global Peace and Consumer Price Indexes were used for comparisons based on MSE, R^2, AIC, BIC and VIF. R Software was used to perform regression analysis, while SPSS 22 was used to perform correlational analysis. The test for significance was made at p-value of 0.01 for the standardized variables. The results from the study show that, LASSO regression produced better models with MSE of 0.7748328, R^2 of 0.1608218, AIC value of 8.683596 and BIC of 12.07329 respectively. Though about 16% of variation was explained. Bridge regression produced better uncorrelated results with VIF of 0.9688117 when q = 2, against LASSO with 1.191642. The correlational result from this study shows that, corruption encompasses different relationships and issues on Human development and Global peace are factors influencing corruption in Nigeria.
Keywords:Correlates; Bridge; LASSO; Corruption perception; Index; Regression; Multicollinearity
Background to the Study
Corruption is one of the most serious challenges to any state’s economic and financial growth and stability. It also has an impact on its people’s standard of living. Factors related to corruption may define the level of perceived corruption. Analysing the origins of corruption and estimating its volume is required for proper response and counteraction actions..
Corruption is a complicated social phenomenon, with different motives resulting from interactions at the small, intermediate, and large levels of corrupt behaviours [1].
However, as data quality and availability have improved, empirical research on corruption has been conducted since the late 1990s in order to design more focused and effective anti-corruption measures to combat corruption. This study aims to gain a better knowledge of relationships as well as identify the most critical factors influencing the Corruption Perception Index. This increases the likelihood of identifying the parts of the economy and social environment that are most vulnerable to corruption.
It is also critical to compare the efficacy of available data in predicting corruption risk in the country, utilising correlation and regression analysis, which will increase the reliability of analytic results, prediction, and anti-corruption actions. Thus, the purpose of this research is to see if Human Development, Global Hunger, Global Peace, and Consumer Price Indexes have any effect on the impression of corruption.
[2] discovered that corruption is socially learnt, and that the majority of people who engage in corrupt activity do not perceive it as bad. This implies that individuals’ participation in corrupt actions is more complicated than a simple decision between ethical and unethical behaviour. Despite the negative externalities of corruption, many engage in it for the personal rewards it delivers. While self-interest is a major motivator for people to engage in corruption, his research reveals that corruption may be motivated by a variety of other causes. Much research compared ridge regression to other regression approaches on medical data [3,4] but not on the corruption perception score.
Cheolwoo P [5] found that bridge regression adaptively determines the penalty order from data and produces adaptable solutions in a variety of circumstances. The numerical analysis reveals that the suggested bridge estimators outperform other penalised regression methods such as the ridge, lasso, and elastic net in a variety of situations. The numerical examples demonstrate that the suggested group bridge estimators outperform other current approaches.
Herawati N [6] conducted research on Regularization of Multiple Regression Methods to Address Severe Multicollinearity. They used Monte Carlo data simulation to compare the performance of the Ordinary Least Square (OLS), Least Absolute Shrinkage and Selection Operator (LASSO), Ridge Regression (RR), and Principal Component Regression (PCR) methods in dealing with severe multicollinearity among explanatory variables in multiple regression analysis.
The study simulated a collection of data with sample sizes of n = 25, 50, 75, 100, and 200 that has severe multicollinearity across all explanatory factors with a (ρ = 0.99). The amount of multicollinearity in the data set was assessed using the Variance Inflation Factor (VIF), and the AIC values of OLS, LASSO, RR, and PCR methods were compared.
Cross-validation is used to calculate the eigenvalue of RR and LASSO. The results suggest that the RR and PCR methods can overcome extreme multicollinearity. In contrast, even if LASSO outperforms OLS, it does not handle the problem particularly well when all variables are highly correlated. Overall, PCR outperforms all other methods for estimating regression coefficients on data with extreme multicollinearity.
Methodology
Research design/ Data collection
Microsoft Office Excel 2010 was used for data entry, computation, coding, and standardization of the data sets. To test for the presence of multicollinearity, Minitab 19 was used to generate VIF for the data sets. Correlational analysis was performed using SPSS 22, and the degree of relationship was established using Pearson and Spearman correlation coefficients. R software was used to analyze the data. MSE, RMSE, R2, AIC, and BIC from the regression analysis were used to compare the best model fit between the Bridge and LASSO regression models. The VIF test was used to determine the degree of multicollinearity in regression models. The data sets were fitted to determine the most effective regression strategy for dealing with multicollinearity. A set of secondary data from 2008 to 2020 was used for comparison and data analysis in this study. Transparency International data on the Nigerian Corruption Perception Index, Country Economy data on the Human Development Index, Knoema data on the Global Hunger Index, Knoema data on the Global Peace Index, and World Bank data on the Consumer Price Index. Appendix 1 contains the data used in the investigation.
Spearman correlation coefficient: The Spearman Coefficient of correlation, 𝜌 is a measure of the closeness of association between two or more ordinal variables and is given by,
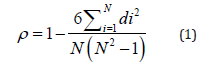
Where di’s are the rank deviation, and N is the number of observations.
The hypothesis for a Spearman correlation can be stated as
follows:
H0: There is no association between corruption perception
index and its correlates.
H1: There is an association between corruption perception
index and its correlates.
Pearson correlation coefficient: The Pearson coefficient of
correlation is given by,
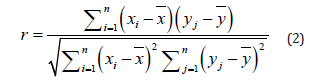
where x ̅ is the mean of variable 𝑥 values, and y ̅ is the mean of variable 𝑦 values.
LASSO regression
When (regularisation parameter) is big, the Least Absolute Shrinkage and Selection Operator (LASSO) regression produces a model in which some coefficient estimates are exactly equal to zero. Hence, the LASSO regularisation does variable selection in addition to regularisation, making the model easier to grasp [7].
The LASSO regression model is defined as the addition of a regularisation factor to the multiple regression model. v =δ0 +δ1 w1 +δ2 w2 +δ3 w3+δ4 w4 +ε +λΣ|δ1 +δ2 +δ3+δ4 | > 0,

where is the regularization term. This regularization term adds a penalty to the multiple regression model which minimizes the SSE. Thus, SSE is expressed as,

Where t is the quality that controls the amount of shrinkage in the estimation of coefficients of LASSO with t ≥ 0
Bridge regression
The Bridge regression estimator is more general than both the Ridge regression estimator and the LASSO regression estimator because it uses a penalty to reduce the SSE. So, the bridge regression method is a way to estimate parameters and choose variables all in one minimization problem. The bridge estimator works well to find large, moderate, and zero covariate effects that are not zero. But it punishes very small coefficient values too much. So, when > 1, the bridge regression method makes the regression coefficients smaller, but it doesn’t let you choose which variables to use. So, when gets bigger, the shrinkage gets bigger along with the size of the regression parameters that are being estimated [8].
Bridge regression model can be stated as adding a regularization term to the multiple regression model

where is the regularization term. This regularization term adds a penalty to the multiple regression model which minimizes the SSE. Thus, SSE is expressed as,

where q is a positive parameter representing the turning constant that controls the amount of shrinkage. 𝛾 is the shrinkage parameter.
Results and Discussion
Correlational analysis results/
From (Table 1), we can see from the spearman’s correlation coefficients for the indices that there is strong correlation between Human Development index and Global Peace index with correlation coefficient of 0.774 respectively. This is significant at 0.002. We also see a weak correlation between corruption perception and human development with correlation coefficient of 0.306 and significant at 0.309, between corruption perception and global peace indices, with correlation coefficient of 0.316 and significant at 0.292 respectively.
Table 3.1: Spearman’s Correlation coefficients for the indices.
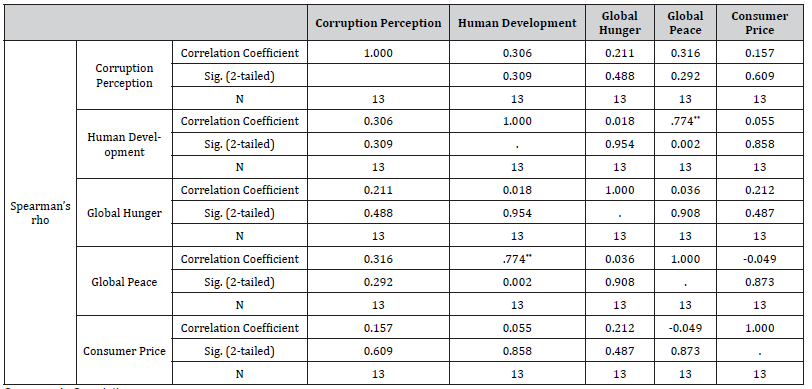
We can say again that from (Table 2), that there is strong correlation among global peace and human development with Pearson coefficient of 0.943 and covariance of 0.944. Global peace index has a moderate association with corruption perception index, with coefficient of 0.515. Whereas consumer price, global hunger and human development indexes have a weak correlation with corruption perception index with Pearson coefficient of 0.216, 0.303 and 0.385 respectively..
Table 3.2:Pearson’s Correlation Coefficients for the indices..
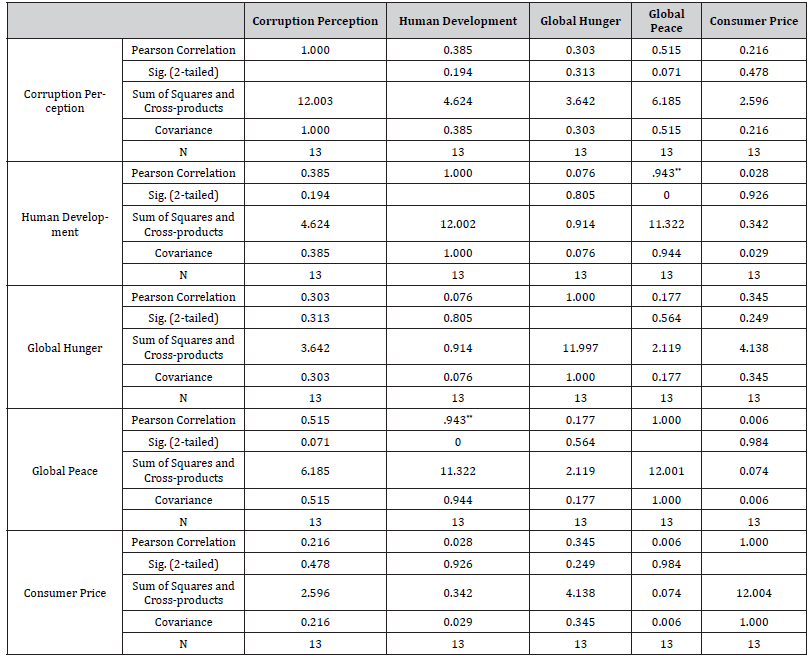
Pearson’s Correlations
Regression Equation for Correlates of Corruption Perception Index Corruption Perception = 0.000-0.912Human Development + 0.058 Global Hunger +1.364 Global Peace + 0.214 Consumer Price (7) From Table 3 above, multiple regression analysis results for Correlates of Corruption Perception Index, we see that ‘Human Development Index and Global Peace Index’ have high VIF values of 10.18, and 10.49 respectively, indicating the presence of high multicollinearity among these independent variables. Thus, the strong correlation between those independent variables means that they can be predicted byother independent variables in the data set. Therefore, we then proceed to use LASSO and Bridge regression analysis techniques to solve the problem of multicollinearity among these data sets.
Table 3:Multiple Regression Analysis Results.
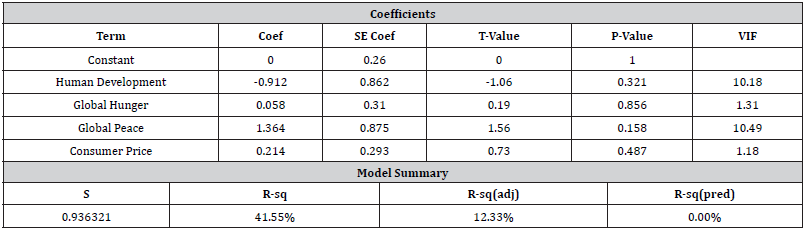
Discussion of Findings
Comparing the best regression strategy to handle the problem of multicollinearity between LASSO and Bridge regressions, Table 3.4 reveals that Bridge regression gave superior results, with a VIF of 0.9688117 for q = 2 and 0.9999519 for q = 1. Bridge regression with q = 2 yielded superior results to bridge regression with q = 1. Bridge regression was conducted using the restricted LASSO (q = 1) and restricted ridge (q = 2) models given by [9]. However, LASSO solved the multicollinearity issue with a VIF of 1.19164. Also, in our comparison of the best model fit, LASSO regression performed better, with MSE values of 0.7748328, AIC values of 8.683596, and BIC values of 12.07332 correspondingly. This is consistent with [10] assertion that the LASSO l 1 penalties are beneficial for fitting a wide variety of models because they do both variable selection and shrinkage. According to the R2 for LASSO regression, approximately 16% of our variance is explained by the model. Consequently, the numerical results of the investigated data set indicate that Bridge regression gives adaptable solutions to multicollinearity in many circumstances. Thus, demonstrating greater performance over LASSO regression, which was also the conclusion of [5].
Summary and Conclusion
Summary
According to our findings, there is a strong correlation between human development in Nigeria and the state’s ability to achieve world peace. We also discovered a slight link between perceptions of corruption, human development, and global peace. Thus, Human Development and Global Peace are factors that influence the impression of corruption in a nation. Again, it was discovered that the issue of multicollinearity in regression analysis can be resolved utilising both the Bridge and LASSO regression procedures. This leads to improved model fitting and more accurate prediction outputs. Therefore, Bridge regression is a superior method for addressing the issue of multicollinearity. LASSO regression performed better than Bridge regression when comparing which model gave the greatest model fit for improved prediction and accurate outcomes. This study’s R Software-based analysis demonstrates that the utilized regression approaches can be used to improve the precision of estimations of regression coefficients and generate superior regression models.
Conclusion
We were able to determine the linkages and influences of various indices on the corruption perception index as a result of this study. Our knowledge of the interrelationships between these indices can be utilized to identify the parts of the economic environment most susceptible to corruption and to examine the causes that contribute to it. Similarly, we were able to demonstrate the most effective regression strategy for dealing with multicollinearity in multiple regression analysis. Understanding LASSO regression and Bridge regression techniques can inform how best they can be used by Statisticians and other Researchers to solve the problem of multicollinearity and in addressing overfitting in model building. This, in turn, can aid in the interpretation of data set analyses, the improvement of regression coefficient estimates, and the prediction of possible response behaviours.
Acknowledgement
None.
Conflict of Interest
No conflict of interest.
References
- Bicchieri C, Ganegonda D (2017) Determinants of corruption: A Socio-psychological Thinking About Bribery, Neuroscience, Moral Cognition and the Psychology of Bribery. Cambridge University Press.
- Tavits M (2010) Why do people engage in corruption? The case of Estonia. Social Forces 88: 1257-
- Ogoke UP, Nduka EC, Nja ME (2013) A New Logistic Ridge Regression Estimator Using Exponentiated Response Function. Journal of Statistical and Econometric Method UK 2(4): 161-171.
- Ogoke UP, Nduka EC, Nja ME (2015) Bipolar Disorder Investigation Using Modified Logistic Ridge Estimator. International Organisation of Scientific Research (Journal of Mathematics) India 11(1): 12-15.
- Cheolwoo P, Young Y (2011) Bridge regression: adaptivity and group selection. Journal of Statistical Planning and Inference 141(11): 3506-3519.
- Herawati N, Nisa K, Setiawan E, Nusyirwan T (2018) Regularized Multiple Regression Methods to Deal with Severe Multicollinearity. International Journal of Statistics and Applications 8(4): 167-172.
- Melkumovaa L, Shatskikhb S (2017) Comparing Ridge and LASSO estimators for Data Procedia Engineering 201: 746-755.
- Olcay A (2015) Penalized MM Regression Estimation with Penalty: A Robust Version of Bridge Regression. A Journal of Theoretical and Applied Statistics 50(6): 1236-1260.
- Bahadir Y, Mohammad A, Fikri A (2019) Penalized Regression via the Restricted Bridge Estimator. Soft Computing 25(2): 8401-8416.
- Tibshirani R (2011) Regression Shrinkage and Selection via the Lasso: A Retrospective. Journal of the Royal Statistical Society: Series B (Statistical Methodology) 73(3): 273-282.
-
Ogoke Uchenna Petronilla* and Nduka Ethelbert Chinaka. Comparative Analysis of Lasso and Bridge Regression Using Corruption Perception Index and Its Correlates in Nigeria. Annal Biostat & Biomed Appli. 5(1): 2023. ABBA.MS.ID.000604..
Correlates, Bridge, LASSO, Corruption perception, Index, Regression, Multicollinearity
-
This work is licensed under a Creative Commons Attribution-NonCommercial 4.0 International License.